Researchers are embarking on a pioneering endeavor to integrate an innately human characteristic, which is “uncertainty,” into machine learning systems. This venture offers potential benefits in enhancing trust and reliability in human-machine collaborations.
(Photo : Tung Nguyen from Pixabay)
Integrating Human Error in Machine Learning
Artificial intelligence (AI) systems often encounter challenges in grasping human error and uncertainty, especially in scenarios where human feedback influences the conduct of machine learning models.
Numerous of these systems are designed under the presumption that human input remains consistently precise and conclusive, neglecting the practicality of human decision-making that encompasses occasional errors and varying levels of assurance.
This collaborative effort involving the University of Cambridge, The Alan Turing Institute, Princeton, and Google DeepMind aims to address this problem between human behavior and machine learning.
By incorporating uncertainty as a dynamic element, this research endeavors to amplify the effectiveness of AI applications in contexts where human-machine collaboration is pivotal, thus mitigating potential risks and bolstering the dependability of these applications.
The researchers modified a renowned dataset for image classification to accommodate human feedback and quantify the extent of uncertainty linked to labeling specific images.
Significantly, this investigation illuminated that training AI systems using uncertain labels could enhance their competence in handling indeterminate feedback. However, it also underscored that the introduction of human involvement could result in a decline in the system’s overall performance.
Read Also: AI Language Models Like ChatGPT Exhibit Political Biases, New Study Finds
‘Human-In-the-Loop’
The concept of “human-in-the-loop” machine learning systems, designed to incorporate human feedback, holds promise in situations where automated models lack the capacity to make decisions independently. However, a critical question arises when humans themselves grapple with uncertainty.
“Uncertainty is central in how humans reason about the world but many AI models fail to take this into account,” said first author Katherine Collins from Cambridge’s Department of Engineering.
“A lot of developers are working to address model uncertainty, but less work has been done on addressing uncertainty from the person’s point of view,” Collins added.
Matthew Barker, co-author and recent MEng graduate from Gonville and Caius College, Cambridge, emphasized the need for recalibrating machine learning models to account for human uncertainty. While machines can be trained with confidence, humans often encounter challenges in providing similar assurance.
To probe this dynamic, the researchers utilized some of the benchmark machine learning datasets involving digit classification and classifying chest X-rays and bird images.
While uncertainty was simulated for the first two datasets, human participants indicated their certainty levels for the bird dataset. Notably, human input resulted in “soft labels,” indicating uncertainty, which the researchers then analyzed to understand the impact on AI model outputs.
Although the findings emphasized the potential for improved performance by integrating human uncertainty, they also underscored challenges in aligning them with machine learning.
Acknowledging their study’s limitations, the researchers released their datasets for further exploration, inviting the AI community to expand on this research and incorporate uncertainty into machine learning systems.
The team posits that accounting for uncertainty in machine learning fosters transparency and can lead to more natural and secure interactions, particularly in applications like chatbots.
They underscore the importance of discerning when to trust a machine model and when to trust human judgment, especially in the age of AI. The team’s findings will be presented AAAI/ACM Conference on Artificial Intelligence, Ethics and Society (AIES 2023) this week.
Related Article: AI-Generated Photos Pose a Threat to Democratic Processes, Experts Warn
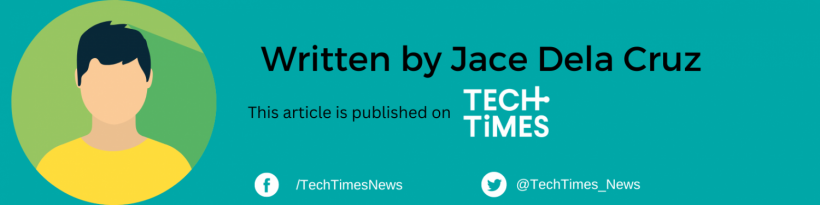
ⓒ 2023 TECHTIMES.com All rights reserved. Do not reproduce without permission.